Adaptive Tests: The First Step to Providing Personalised Education

ADAPTIVE ASSESSMENT IS A MACHINE LEARNING TECHNIQUE WHICH ACCURATELY QUANTIFIES THE PROFICIENCY OR KNOWLEDGE LEVEL OF THE EXAMINEE
“Until we get equality in education, we won’t have an equal society.”
-Sonia Sotomayor
(Asst. Chief Justice, Supreme Court, USA)
Education can help every mind grow in a unique way, thus providing equal opportunities to all learners for the development of society. It is, as the acclaimed educational reformer Horace Mann says, the ‘the great equalizer’ and ‘the balance wheel’ of the society, for it brings everyone to the threshold of new possibilities via personal initiative. However, the self-driven initiative fails when the learning is designed to fit everyone in the same mould, instead of catering to the unique needs and abilities of individual learners. For education to meet the requirements of the students, it is necessary for the instructor to know precisely where the individual learner stands at the beginning of the academic course and to measure the gaps through the course of learning. Assessment is the best method to carry this out.
Unfortunately, fixed form tests, with the same set of questions for all examinees, do not have the ability to adjust to individual achievement levels, rather they serve the average students best and give less precise scores for students at the extremes of the performance continuum. These tests do not give an accurate picture of what the learners know or don’t know, and what kind of instructions the students need to learn new concepts. These are efficiently measured by Computerized Adaptive Tests (CATs), a machine learning application which accurately quantifies the proficiency or knowledge level of the examinee.
THEORIES OF ADAPTIVE TESTS
There are three major theories on which adaptive assessments are based: Item Response Theory (IRT), Testlet Response Theory (TRT) and Knowledge Space Theory (KST). The simplest way of implementing an adaptive test is by using the Item Response Theory. In it’s most famous form, called 2-PL (Two-Parameter Logistic) IRT, each question has two parameters: difficulty and discrimination. These parameters are assigned to the questions by a machine learning algorithm based on real responses of students to these questions as a part of a normal static test. Since the parameters are based on data and not on a subjective opinion of a person, they are more reliable and accurate.
Once the parameters are assigned to the questions, the computer program picks questions of average difficulty level from the question pool. After a question is answered by the student, the program re-calculates the student’s ability based on whether the answer given by the student is correct or not. If it’s correct, student’s ability estimate goes up and the program picks tougher questions. If it’s incorrect, student’s ability estimate goes down and the program picks easier questions. In this manner, the program keeps adjusting the difficulty of the question to suit the level of the student. The program keeps throwing questions until the ability of the student is measured to a satisfactory level. It generally picks questions with a higher discrimination value as they help end the test faster by giving more information about the ability of the student.
Testlet Response Theory (TRT) is a relatively new approach to item analysis and test construction that uses testlets — groups of items administered as a single unit, often having a common motive. IRT models for certain kinds of questions, such as reading a comprehension passage and answering a related series of questions, hinder the adaptive test from giving precise scores since all items are considered independent of each other. TRT-based tests resolve this issue by estimating local dependency within testlets for each testlet and for each examinee. Therefore, the same set or number of items within a testlet are not needed to be administered for all examinees. These tests are aimed at assessing an examinee’s understanding of literary pieces, case studies, maps, musical pieces, etc., which makes it more efficient for subjects such as Humanities and Social Studies.
These approaches have been used widely in tests such as GRE, TOEFL and SAT developed by the Educational Testing Service and GMAT conducted by the Graduate Management Admission Council for admissions to universities in the USA. India’s National Testing Agency (NTA) has also proposed the use of adaptive assessments to conduct entrance examinations for higher educational institutions — JEE Main, NEET UG and NET— so as to bring higher reliability and for assessing the aptitude of students.
Next Education has implemented adaptive tests based on IRT for CBSE Maths and Science from grades 6 to 10 to gauge the chapter-wise level of knowledge of a student. The adaptive tests are available for schools on NextLearningPlatform. They’re also available for students at our self-learning solution, LearnNext. The high accuracy of adaptive tests was possible because of millions of responses collected anonymously since 2007. The adaptive tests in LearnNext allow learners to evaluate themselves at any time and place with a vast pool of 60,000 questions. Moreover, our award-winning in-house content consisting of HD animated videos and digital books available on LearnNext/NLP will allow the students to independently bridge the gaps in their learning.
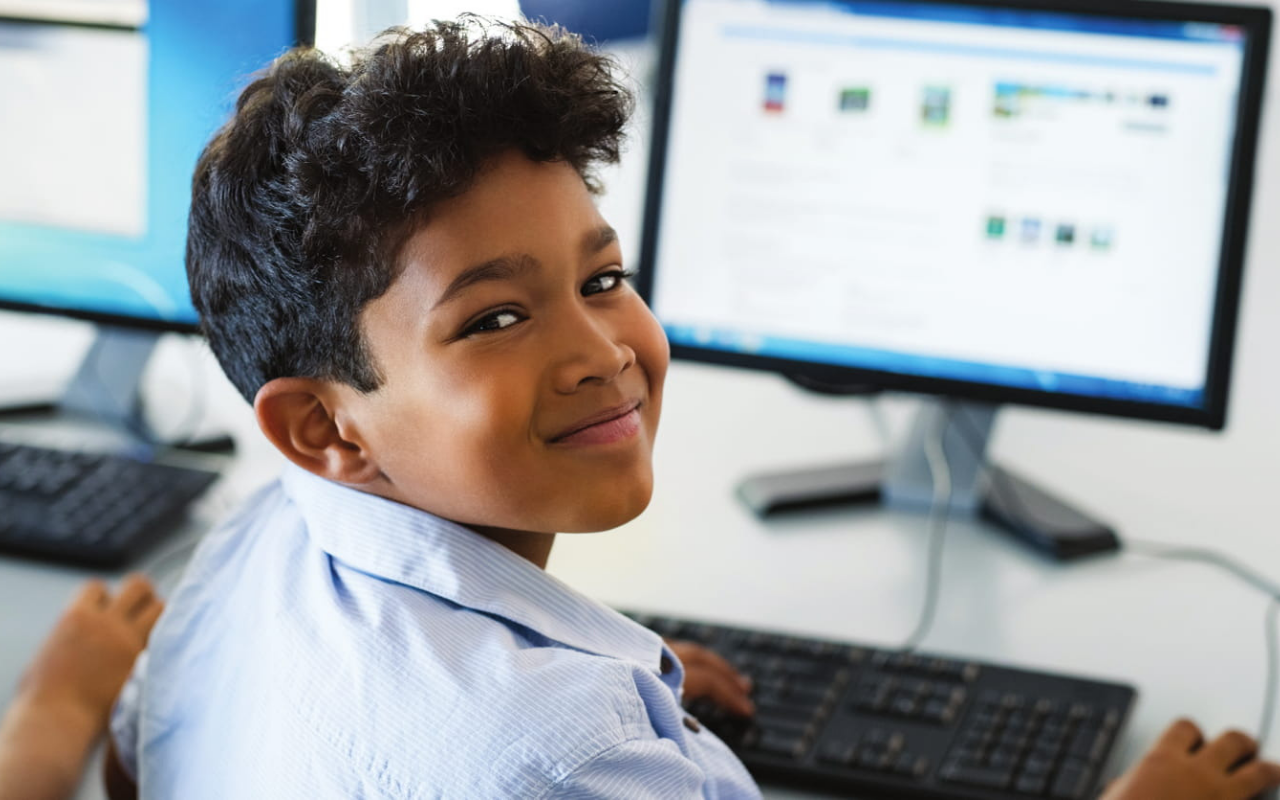
Knowledge Space Theory (KST) is a theory of mathematical psychology that accurately measures the knowledge state of a learner as a subset of all concepts that are possible to be known by an individual. If it is assumed that there are certain knowledge components to learn, a dependency graph follows specifying which components need to be mastered before learning a particular component. This helps to create possible combinations of various knowledge components which indicate the knowledge states of the learners. For example, in the diagram given below, mastering concept ‘a’ means that the learner knows nothing else, but mastering concept ‘e’ means that the learner has mastered the concepts ‘a’, ‘b’ and ‘c’ and the knowledge state can be represented as {a,b,c,e}.
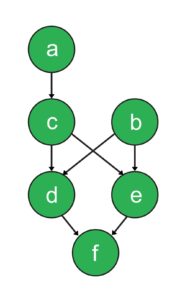
Assessments based on this model can specify what learners have already mastered and what they are ready to learn. Next Education is currently working on providing such assessments for CBSE Maths and Science for grades 6 to 10 which will be ready by December 2018. This model has been implemented in Assessment and Learning in Knowledge Spaces (ALEKS), an online tutoring and assessment programme in the UK, which is used by millions of K-12 learners today.
Benefits of Adaptive Tests
- Adaptive tests have a higher degree of precision in generating scores. They help distinguish between students who score equally in traditional tests, providing uniformly precise scores for all students.
- These tests adapt to the ability of the examinee, reducing the duration of examination by 50 to 75% compared to standard fixed tests. This not only saves time, but also the cost of examinee seat time.
- Adaptive tests provide questions with the difficulty level suited to the current estimate of the examinee’s proficiency. Therefore, it acts as a morale booster, since the chances of discouragement or boredom are reduced.
- Adaptive tests eliminate the chances of cheating as students get different questions.
- Examinees can continue practising and get questions of varying difficulty levels every time they take the test.
- Aided by its vast question pool and personalised difficulty levels for every examinee, adaptive tests reduce the pressure on teachers of creating tests suited to the abilities of individual learners.
- Adaptive reports enable teachers to analyse class performance with respect to global test-takers and identify gaps in learning. Instructors can receive detailed insight on a student’s mastery across grade levels, rather than within a specific grade level. This will help them design personalised learning paths for individual learners.
Thus, with the help of adaptive tests, it is possible to streamline the process of assessment and evaluation of learners, to measure the disparities in learning and to bridge the gaps easily, which will then fulfil the promise of education as an equalising force for the society.